Intent: understanding what a test really does
Testing involves following a set of steps to get a result. But you must understand the intent behind the test. Here, we explain why this is also true for AI.
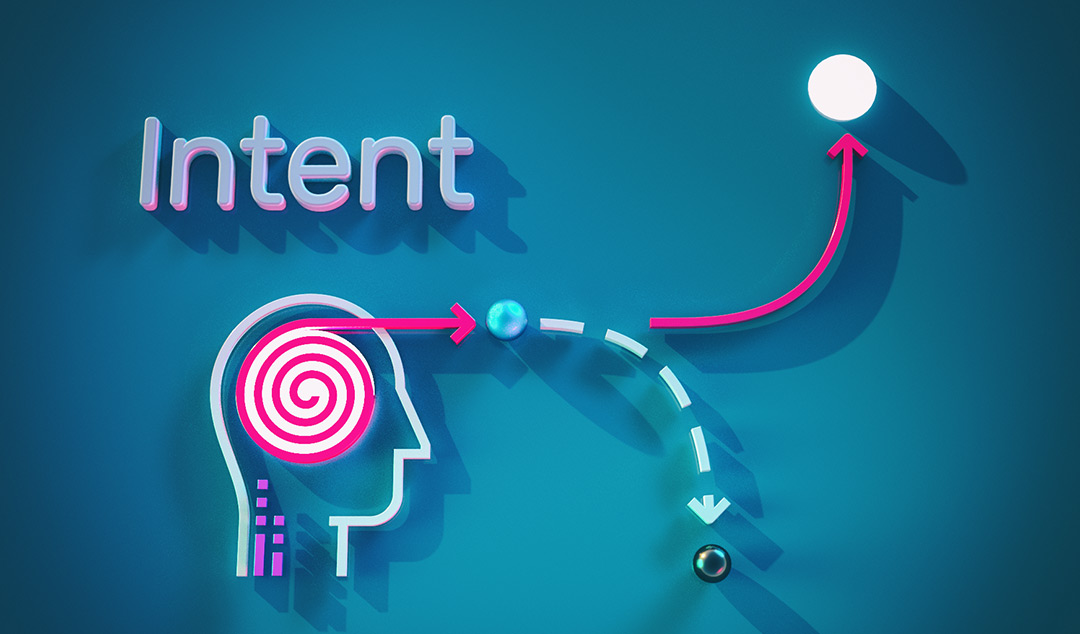
Testing is about following a set of test steps and getting a result. So, creating test automation just requires converting those steps into a script. Or at least, that’s what some people seem to think. In this blog, we explain why it is also vital to understand the intent behind each step.
Introduction
Intentions matter. As humans, we usually don’t act without having some intention about the outcome! If you intend to make coffee, you first turn on the coffee machine. As a human, you understand that turning on the coffee machine indicates the intention to make coffee. This is as true of interactions with UIs as it is of everyday life. Indeed, good UX design is all about making it easy for a user to indicate their intentions.
Intentions are also really important when you are testing. In the old days, test plans were a set of steps for a human to follow when testing your product. Plans were written by humans for humans. The plan could ask the user to “login with the test account” and know that the human tester would understand what to do. More importantly, the human tester would understand why they needed to do it. In other words, they would understand the intent behind the test. Namely, to test whether the login works.
For traditional test automation, using simple test scripts, the test engineer needs to understand the intent of the test. This is because the script will just dumbly follow the instructions given to it. If a page takes too long to load, the script will still try to interact with it unless you add delays. If a button is swapped, the script will just click the wrong button, triggering a failure and the need for test maintenance.
What is intent and why does it matter?
Dictionary definitions for intent are pretty much circular. Merriam Webster defines it as “a usually clearly formulated or planned intention”. Look up ‘intention’ and you find “what one intends to do or bring about”. So, for the sake of clarity, in this blog, we use intent as follows.
Intent (noun): The aim behind a specific action or set of actions.
This is probably easiest to understand if you think about creating a test from scratch. Typically, you start with a user journey from the product team. This user journey takes the user from one state in the application to another, for instance, it might involve updating the user’s personal details. Ergo, the intent here is to update the user’s details. This user journey is then converted into a set of detailed steps in a test plan. Taken as a whole, this test plan still makes it clear what the intent is. However, once you hand that test plan to a Test Engineer, the intent may start to get blurred. And of course, a computer that runs the test has no idea what the intent is at all!
“So what?” I hear you ask
You may think it is OK that the computer doesn’t understand what is going on. After all, a human is in charge of creating the test script and analyzing the results. The problem is, over time both tests and UIs evolve. New engineers join the team and have to update or maintain existing tests. Tests become obsolete as new tests are created. You need a proper understanding of the intent of a test in order to know if a new test duplicates it. Likewise, you need to understand if a step becomes redundant due to a change in the user journey. The problem is, test engineers can get too focused on just repairing tests that have failed.
Intent in test automation
Test automation typically involves getting a computer to select an element on the UI, interact with it in some way, and then check the result. In other words, a test is simply a set of actions the computer should perform. The computer doesn’t care what a button does. It just cares that the script asks it to click on it. Having interacted, the script then tells the computer to check the result. Typically, this involves checking to see if specific (new) elements have appeared.
A simple example
Let’s take one of the simplest possible examples, namely the login user journey. Almost all websites have some form of login. For simplicity, we will look at the Facebook login. For the human creating the script it is pretty obvious what the intent is:
from selenium import webdriver
But what about the computer running the test? As far as the computer is concerned this test says nothing about intent. It just tells it to:
- Load a given web page
- Look for a text field on that page and enter some data
- Find another text field and enter some different data
- Locate a specified button and click it
Obviously, this is a toy example. When a human comes to inspect this script they can still tell this is a login script. But what isn’t clear is whether this test is meant to pass or fail. Are the user details ones for a valid login or not? After all, every good test plan should test the unhappy path as well as the happy one!
Why intent is a hard problem for AI
Here at Functionize, we have developed an AI-powered test automation framework. Our aim is to simplify the process of test creation and test maintenance in order to boost your team’s productivity and eliminate test debt.
The starting point is to use our smart recorder, Architect, to create your test. This Chrome plugin allows you to step through your test on screen. The process uses a number of AI paradigms including machine learning, deep learning, and computer vision. It combines these to build up a detailed model of the UI based on all the tests you create. In the background, our system is also trying to learn the intent behind your test. Both of these are vital in order for the resulting test to be robust.
Using this approach, the system is very good at learning the outcome of events. E.g. if it clicks the login button, it knows this should result in moving to a new page with a user logged in. However, it doesn’t really understand what the new page is. It just knows that it is what happens. This means that while the AI is learning it can become confused. To an AI any page that loads will seem to be a valid outcome unless it is taught that it’s wrong.
What happens if the intent isn’t obvious?
Understanding the intent of the test is integral to understanding what the outcome should be. This is something the AI simply can’t do on its own. Usually, if the test is well-structured, this isn’t a major problem. However, things can go wrong. The wrong page might load, or there may be an unexpected popup. Hopefully, the person recording the test will know what to do, but that isn’t always true. And this problem is exacerbated when you are dealing with specialist systems where domain knowledge is critical.
How to add in the missing context?
One of the hardest problems we faced was how to teach our system about the intent behind your test. How could we add more context to tests? Our solution is to allow you to provide contextual information when creating tests. In Architect, this information is added using verifications.
Verifications allow you to tell the AI exactly what should happen. They take many forms. For instance, you might tell it which page should load next. Or maybe tell it what entries are in a given menu. The important thing is to use enough of these verifications to help steer the AI in the right direction. Then, if things change in your UI, the system will be able to use its knowledge to work out what happened. The upshot is, you will spend almost no time doing test maintenance. Instead, you will be able to focus on creating more tests and analyzing the test outcomes.
What next?
Maybe you are thinking about updating your test automation? Or perhaps you realised how much time your team now spends on test maintenance? You might just be intrigued to see what best-in-class AI-powered automation looks like. Whatever the case, book a demo with us today.