What are canary launching and dark testing?
A review of canary testing and dark launching, two popular approaches for testing new features and performance in complex applications
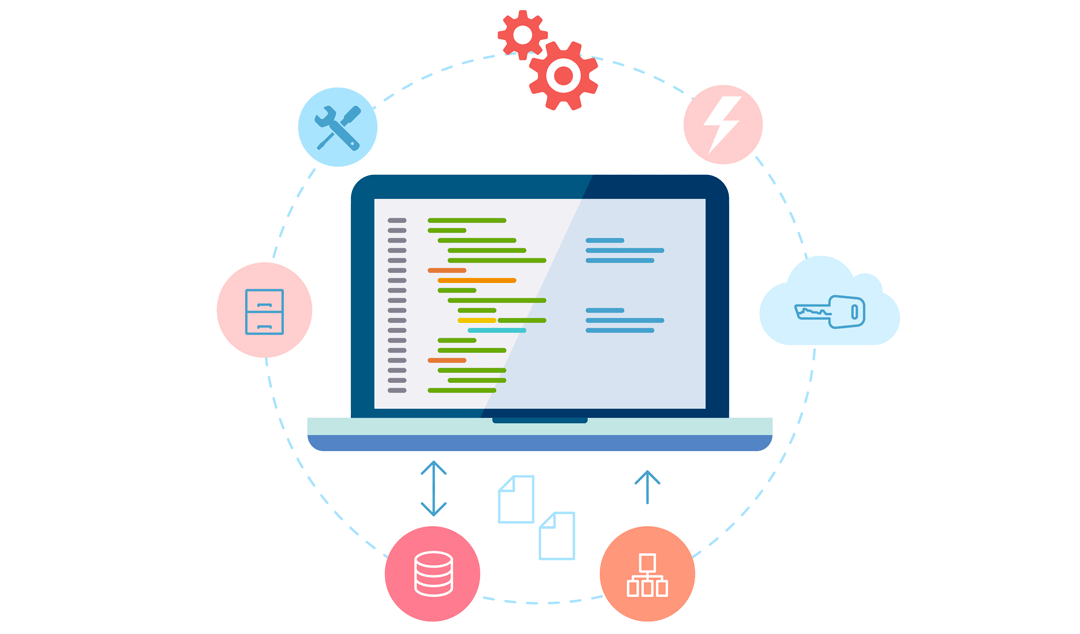
Your software development teams want to release new product features frequently, but without endangering established production systems or confusing users who are familiar with the existing customer experience. A common answer to the challenge is dark launching and canary testing. Learn what these practices are and how they can help your organization -- and your users!
As Agile development becomes more commonplace, companies are increasingly moving away from red-letter day launches for new software features. Nowadays, it’s business-as-usual to update applications regularly, using platforms such as AWS or Google Cloud. So, how do they ensure these features are robust and work well? This is where canary testing and dark launching help programmers and testing teams.
Originally, most microcomputer software was delivered as stand-alone applications that were installed on a user’s computer. Developers only released updates periodically -- usually measured in months, if not years. Before each update, the development team would invest heavily in testing the software for performance, security, and general quality. Doing so involves creating a release candidate and testing it against a full set of test plans.
The organization might need to release updates between major releases. These typically are small changes that can be tested in the traditional manner.
However, a different model emerged. It’s rare for software to be released in infrequent release cycles, anymore, and most applications are web-based or work on mobile devices. To stay ahead, developers need to constantly update applications; users expect frequent functional improvements. This means that development teams need to adopt a continuous integration and continuous delivery (CI/CD) methodology, which requires an evolution in software testing.
Instead, you need to turn to a more Agile approach, which includes canary testing and dark launching.
What is canary testing?
Traditional testing relies on an all-or-nothing product launch. The development and software testing departments do their level best to check the software for all important attributes, and then release it to the wild -- so that all users have access to the new version.
In contrast, with canary testing, the development team solicits a small number of users as test subjects for the new code. The term comes from the practice of sending canaries down into mines to detect the presence of methane and other toxic gases.
The premise is that the development team uses the software’s users as “canaries” who can detect defects in a new release, whether in functionality, stability, scalability, performance, or some other aspect of software that gives users joy. The aim is to compare the test users against users on the older code.
You set up the canary system by launching a set of back end containers or servers running the new code. As new users arrive, the load balancer deflects a percentage of them to these “canary servers.”
To discern how well (or poorly!) the new version performs, the development team, test team, and DevOps carefully monitor the canary servers to identify issues. For instance, a developer might monitor the compute load, and compare it to the servers running the old code. If the load increases substantially you know that’s a potential issue. Equally, if you see a much higher rate of I/O that might also indicate an issue.
Because only a subset of users are affected, this real-world testing process doesn’t cause problems for everyone. If the testing team spots any issues, it’s easy to roll things back. It is as simple as redirecting all arriving connections back to the old servers and migrating the customers off the canary servers.
How many people should be in a canary test? It varies, of course, but a typical canary test assigns about 5% of users to the new code. Then, if there are no issues, the DevOps team can steadily ramp up the user percentage until everyone is on the new code.
How to run canary tests
Developers and tester You can easily implement and automate canary testing, with the help of using tools such as Spinnaker to assign a suitable percentage of users to the new code.
According to its technology blog, Netflix further refines this process. Netflix doesn’t compare the performance of the canary servers with its existing production servers. Instead, the company creates new instances of both the existing servers and the canary servers. This so-called baseline cluster is the same size as the canary cluster. The canary cluster’s performance can be compared directly with the baseline. This means the results are compared against a clean setup with no potential issues caused by long-running processes in the production cluster.
When you engage in canary testing, be fully prepared for possible impact from the new code. It may be that the new changes are known to increase the I/O in the system, in which case seeing increased I/O does not indicate a problem. In other words, carefully identify which metrics matter for each test and then define the acceptable parameters. Of course, some issues such as crashes, stuck processes, or timeouts are almost always signs of a problem with the new code.
Dark launching
Dark launching is similar to canary testing. However, where canary testing largely looks at an application’s back end, dark launching assesses user response to new features in the application’s front end. It’s more about user interface testing than system throughput.
The idea is that rather than launch a new feature for all users, you instead release it to a small subset. Usually, these users aren’t aware they are testing the new feature; often, nothing highlights the new functionality -- hence the term dark launching.
How to do dark launches
These features can be toggled on or off using feature flags.
First, ensure the deployment system can use feature flags. This may require a new approach from your developers. But most modern applications are inherently modular like this.
To run a test, the product team selects a feature to turn on for a set of users. The UX instrumentation in your application can monitor user response. Some things, like whether she actually finds the new feature and whether she interacts with it, can be measured directly. Others have to be measured indirectly, such as whether the new functionality seems to improve the actual experience or if it increases revenue. There isn’t much new in this, conceptually; this is what the product manager does to assess application performance. The only difference is that the development team is looking at the performance of a single new feature.
Before the development team decides to adopt a dark launching approach for feature testing, ensure that every application feature can be toggled on or off. This allows you to use an API to enable the relevant set of features to test. This approach also allows you to do classic A/B testing of users to compare two versions of your new feature.
You can see how commonplace dark launching is by using a popular online application, such as Google Maps. You frequently see new features that may later disappear. This is because the Google team is constantly trying things out to decide what works best. Things that work are rolled out across the entire user base; other features are quietly dropped. Users of Google Maps who live in or near Zurich are particularly likely to see new features since the core development team for Google Maps is based there.
How automated testing helps
You might think that these approaches let the development and testing teams get away with doing less testing s. But, sadly, that isn’t the case. First, because these approaches use your own users as test subjects, you want to ensure no one has a negative user experience. Second, both approaches to test whether new features are good -- appreciated, noticed, used. There’s no point in doing sot if the new features don’t work properly.
That’s where intelligent test automation comes in.
With proper test automation, developers can rapidly complete a full set of regression tests. If you designed them well, the regression tests verify that everything is working.
However, automating an entire test suite is challenging, even if you can afford to hire a large team of test automation engineers. Fortunately, Functionize’s intelligent test agent and new architect greatly simplify test automation. Our suite of tools makes it quicker and easier to create new tests. They speed up the process of running and analyzing test results. And they avoid much of the requirement for test maintenance that is a problem for so many other test automation frameworks.
What’s next
Canary testing and dark launching are widely used for testing new features in complex applications. Canary testing is ideal to test the performance of an application back end; dark launching is for testing new user interface features. Both approaches lend themselves to automation and can even be coupled with AI to produce a completely automated testing approach.
If you’re concerned about how the software performs in production, you may also find it instructive to read our white paper explaining intelligent performance metrics.