Why AI/ML Testing Needs Cloud Infrastructure
AI/ML models require cloud servers to optimize their performance. In this post, we will dive into the reasons why AI technology necessitates the use of cloud servers and the advantages that can be anticipated.
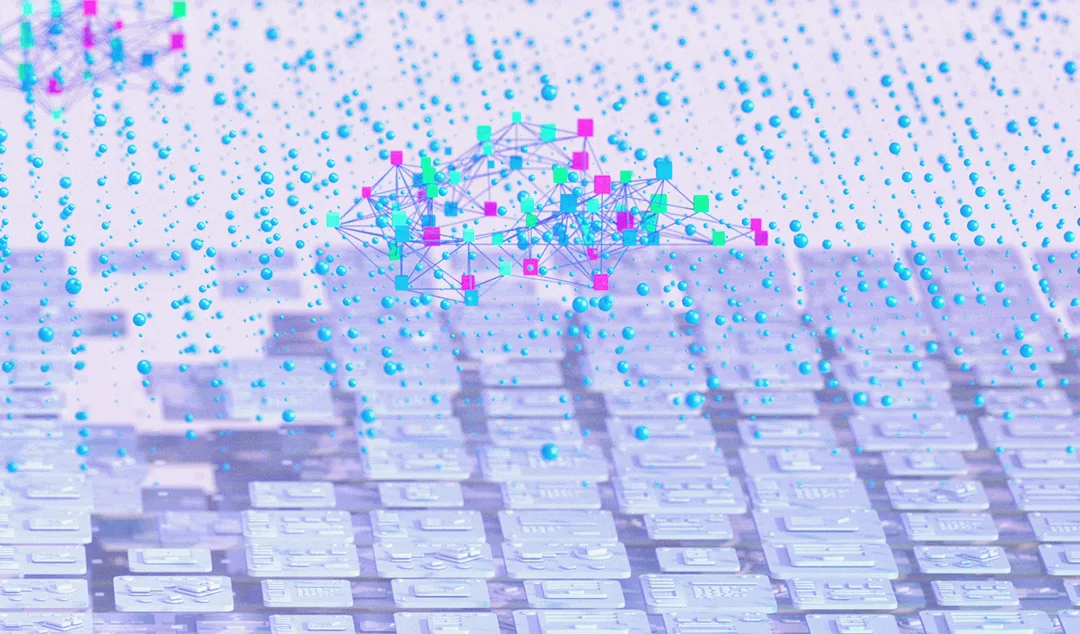
Artificial intelligence (AI) technology has come a long way since its inception. Today, AI is being used in various fields, including finance, healthcare, transportation, and more. The increasing demand for AI has led to the development of several powerful machine learning algorithms and deep learning models.
However, AI models require significant amounts of computational power and memory, which makes running them on local machines nearly impossible.
AI models need cloud servers for efficient performance. In this post, we will discuss why AI technology needs cloud servers and the benefits you can expect.
Scalability
One of the primary reasons why AI models require cloud servers is scalability. AI applications work with large volumes of unstructured data. It’s tricky to manage infrastructure when data is not stored in predefined units of storage. Unstructured data comes with unpredictability. Moreover, AI programs especially need to collect more datasets in the training phase so that they can learn, refine and yield results. This data can run into several petabytes that need to be stored and processed seamlessly. The ability to scale quickly and efficiently is crucial for AI applications.
When you run AI models on a local machine, you are limited by the hardware's processing power and memory. This can lead to performance issues and make it difficult to scale. Cloud servers offer scalable computing resources that can be easily upgraded or downgraded as per the application's requirements. This enables developers to quickly add or remove computing resources as required, ensuring efficient and seamless performance.
Cost-effectiveness
Another significant benefit of using cloud servers for AI applications is cost-effectiveness. The unpredictable nature of AI’s unstructured data also means unpredictable costs. Compounded by the volume of data that’s needed to learn and process, especially in the training phase, AI implementation has the potential to be very costly.
Running AI models on a local machine requires significant hardware investment. You need to purchase high-end CPUs, GPUs, and other hardware to run AI models efficiently. This can be expensive and time-consuming. In contrast, cloud servers offer a cost-effective alternative to running AI models on a local machine. Depending on the stage of the AI implementation, you only pay for the computing resources you use, which can significantly reduce the costs of running AI models.
Collaboration
Teams are now increasingly adopting hybrid work models, with digital assistants, unified communications platforms and advanced productivity tools making collaboration more seamless than ever. Menial tasks that would take days are being completed in minutes, allowing team members to focus on creative and strategic work. These technologies leverage AI for their features, which requires cloud infrastructure.
Cloud servers provide access for AI models to run from anywhere. This is especially important in applications where multiple users in a geographically distributed group need to access AI-enabled features simultaneously. With cloud servers, the AI models can be accessed from any location, making it possible to collaborate easily with remote teams and working wonders with time and productivity.
Maintenance
AI models are prone to model drift, i.e. the drop in quality or performance of an AI model once it has processed real-world data. Training and deploying an AI model with an initial dataset is only the beginning of its lifecycle - to make an AI model effective, it needs to be monitored and maintained to keep them running smoothly, just like any other application.
Running AI models on a local machine requires significant time and effort to keep the hardware and software updated. Cloud servers, on the other hand, offer automated maintenance and updates, making it easy to keep AI models up to date. This ensures that the models are running at peak efficiency and helps avoid any potential downtime or performance issues.
Security
Finally, cloud servers offer enhanced security features that make them more secure than local machines. These features include firewalls, data encryption, access control, and more.
With cybersecurity risks on the rise, cloud providers now have inbuilt security measures that make managing security easier. Security controls are embedded into native cloud applications, and automation makes security processes and solutions scalable. This is crucial for AI deployments which will likely encounter risks compounded by scale along its lifecycle.
The Bottom Line
AI technology needs cloud servers to perform efficiently and effectively. This also applies to quality assurance during design and deployment of AI applications. With AI models designed to continuously learn and adapt, testing AI applications becomes especially complex. QA for AI applications includes testing for training data, configuration data and real-world data.
The scalability, cost-effectiveness, collaboration, maintenance, and security benefits offered by cloud servers make them the best choice for testing and running AI models. As AI technology continues to evolve, cloud servers should remain an integral part of AI infrastructure, providing the computing power and resources required to drive the growth and success of AI applications.
The Functionize Test Cloud
Functionize is a cloud-based test automation platform that offers various testing solutions to help developers and QA teams build and run tests in a scalable and efficient manner. One of the core features of Functionize is its Test Cloud, which provides several advantages to users. This cloud-first infrastructure makes it ideal for testing AI design and deployment.
Here are some of the advantages of using the Functionize Test Cloud:
Scalability: The Test Cloud is designed to be scalable, which means that it can handle large test suites and multiple testing environments. This scalability enables teams to perform tests more quickly and efficiently, without being limited by local hardware resources.
Global Coverage: The Functionize Test Cloud has a vast network of testing locations that are distributed worldwide. This global coverage means that tests can be run in different geographic locations to ensure that they work optimally in all regions. This makes it easier for teams to test their applications across a range of real-world scenarios.
Security: Functionize's Test Cloud is built with security in mind. It uses encryption and secure communication protocols to ensure that data is protected. This high level of security is especially important for teams working with sensitive data or in industries where security is a top priority.
Easy Integration: The Test Cloud is designed to integrate easily with other testing tools and frameworks. This integration enables teams to run tests using the tools they're familiar with and helps to reduce the learning curve when adopting new technologies.
Cost Savings: By using Functionize's Test Cloud, teams can save money on hardware, software, and maintenance costs. The cloud-based solution eliminates the need to invest in hardware, as tests can be run on virtual machines. This can be especially beneficial for small teams or startups that have limited resources.
Continuous Testing: The Test Cloud enables teams to run tests continuously, as part of a continuous integration and deployment (CI/CD) pipeline. This continuous testing approach helps to identify and fix issues quickly, before they become more significant problems.
Get a demo of the Functionize Test Cloud today.